์ผ | ์ | ํ | ์ | ๋ชฉ | ๊ธ | ํ |
---|---|---|---|---|---|---|
1 | 2 | 3 | 4 | 5 | ||
6 | 7 | 8 | 9 | 10 | 11 | 12 |
13 | 14 | 15 | 16 | 17 | 18 | 19 |
20 | 21 | 22 | 23 | 24 | 25 | 26 |
27 | 28 | 29 | 30 |
- Linear Regression
- ๋ฏธ๋์์ ํด์ธ๊ตํ
- Deeplearning
- ๊ตํํ์
- ์ ๋ฝ ๊ตํํ์
- set method
- Python
- Andrew ng
- Machine learning
- 2022๋
- ์ ํํ๊ท
- ์ค์คํธ๋ฆฌ์
- ์๊ทน์ฌ
- m1 anaconda ์ค์น
- ์ด์ฐจ์ ์ง
- ํน๋ณ ๋ฉ์๋
- ๋ฏธ๋์์ ์ฅํ์
- li-ion
- ์ ๋ฝ
- set add
- fatigue fracture
- fluent python
- ๋ฅ๋ฌ๋
- electrochemical models
- anaconda ๊ฐ์ํ๊ฒฝ
- cost function
- ์ฒญ์ถ ํ์ดํ
- gradient descent
- ๋์23์ด
- special method
- Today
- Total
๋ชฉ๋ก๐ ์ด์ฐจ ์ ์ง (11)
Done is Better Than Perfect
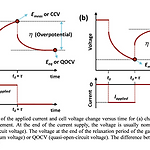
๋ฐฐํฐ๋ฆฌ๋ฅผ ํ๊ดดํ์ง ์๊ณ ๋ฐฐํฐ๋ฆฌ ๋ฌผ์ฑ์ ์ดํดํ ์ ์๋ ๊ธฐ๋ฒ์๋ GITT, PITT, EIS, OCV, CV, dQ/dV ๋ฑ์ด ์์ต๋๋ค. ์ด ๊ธ์ GITT(Galvanostatic Intermittent Titration Technique)๋ฅผ ๊ณต๋ถํ๋ฉด์ ์ ๋ฆฌํ ๋ด์ฉ์ผ๋ก GITT์ ์คํ ์๋ฆฌ๋ถํฐ, GITT๋ก ์ ์ ์๋ ๋ฐฐํฐ๋ฆฌ ์ํ ์ ๋ณด, ์์ ์ด(two-phase) ์์คํ ์์์ GITT์ ํ๊ณ์ ๊ทธ ๋ณด์ ๋ฐฉ๋ฒ์ ์์๋ณด๊ฒ ์ต๋๋ค. ๐ ๐ ๋ชฉ์ฐจGITT๋ ๋ฌด์์ธ๊ฐ?GITT ์คํ ์๋ฆฌGITT๋ก ์ ์ ์๋ ๋ฐฐํฐ๋ฆฌ ์ํ ์ ๋ณดGITT vs PITT: ์ด๋ค ์ํฉ์์ ๋ฌด์์ ์ธ๊น?One-phase vs Two-phase ๋ฐ์์์์ GITT ํด์ ์ฐจ์ดTwo-phase ๋ฐ์์์ GITT ์๊ณก ํ์๊ณผ ๋ณด์ ๋ฐฉ๋ฒ 1. GI..
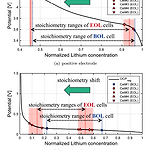
๐ฉ ๋ฐฐํฐ๋ฆฌ์์ Stoichiometry(ํํ์๋ก )๋?๋ฐฐํฐ๋ฆฌ์ ์๋ช ๊ณผ ์ฑ๋ฅ์ ์๊ฐ์ด ์ง๋จ์ ๋ฐ๋ผ ์ ์ง์ ์ผ๋ก ์ ํ๋ฉ๋๋ค.์ด๋ฅผ ๋ฐฐํฐ๋ฆฌ ์ดํ(degradation)๋ผ๊ณ ํ๋ฉฐ, ์ดํ๊ฐ ์งํ๋๋ฉด์ ๋ฐฐํฐ๋ฆฌ ๋ด๋ถ์ ๋ค์ํ ์ ๊ธฐํํ์ ํน์ฑ์ด ๋ณํํ๊ฒ ๋ฉ๋๋ค.ํนํ, ๋ฐฐํฐ๋ฆฌ์ ์ฃผ์ ๊ตฌ์ฑ ์์์ธ ์๊ทน๊ณผ ์๊ทน ๋ด ๋ฆฌํฌ ์ด์จ ๋๋์ ๋ณํ(์ฆ, Stoichiometry)๋ ์ดํ ๊ณผ์ ์์ ์ค์ํ ์ญํ ์ ํฉ๋๋ค. Stoichiometry(ํํ์๋ก )๋ ์ผ๋ฐ์ ์ผ๋ก ํํ ๋ฐ์์์ ๋ฐ์๋ฌผ๊ณผ ์์ฑ๋ฌผ ์ฌ์ด์ ์ ๋์ ๊ด๊ณ๋ฅผ ๋ค๋ฃจ๋ ๊ฐ๋ ์ด์ง๋ง, ๋ฐฐํฐ๋ฆฌ์์๋ ์ ๊ทน(์๊ทนยท์๊ทน) ๋ด ๋ฆฌํฌ ์ด์จ ๋๋์ ์ ๋์ ๋น์จ์ ์๋ฏธํฉ๋๋ค.์ถฉยท๋ฐฉ์ ๊ณผ์ ์์ ์ ๊ทน ๋ด ๋ฆฌํฌ ๋๋๊ฐ ๋ณํ๋ฉฐ, ์ด๋ฌํ Stoichiometry ๋ณํ๋ ๋ฐฐํฐ๋ฆฌ์ ์ ์ ํน์ฑ, ํ์ฐ ์๋, ๊ทธ๋ฆฌ๊ณ ..
๋ฐฐํฐ๋ฆฌ์ ์ดํ์ ๋ฏธ์น๋ ํ๋ผ๋ฏธํฐ๋ค์ ์ํฅ์ ์์๋ณด๊ธฐ ์ํด, ๋ฐฐํฐ๋ฆฌ ์๋ฎฌ๋ ์ด์ ๋ผ์ด๋ธ๋ฌ๋ฆฌ์ธ PyBaMM์ ๊ธฐ๋ณธ ๋ด์ฅ๋์ด ์๋ parameter set์ ๋ถ๋ฌ์ ์ดํ์ ์์(์: Li-plating, LAM, SEI ๋ฑ)์ ์ํฅ์ ๋ฏธ์น๋ ํ๋ผ๋ฏธํฐ๋ค์ ๋ถ๋ฅํด๋ณด์์ต๋๋ค. ๐ฉ PyBaMM ๋ผ์ด๋ธ๋ฌ๋ฆฌ์ ํ๋ผ๋ฏธํฐ ์ ์ฅ OKane2022 parameter set์ ๋ถ๋ฌ์ ๊ฐ์ ํ์ธํ๊ธฐ ์ํ์ฌ Excel ํ์ผ๋ก ์ ์ฅํ์์ต๋๋ค.OKane202 parameter๋ LG M50 cell(NMC)์ ๋์์ผ๋ก ํ ํ๋ผ๋ฏธํฐ๋ก, ๋ ผ๋ฌธ O'Kane et al [1]์์ Chen et al [2]๋ฅผ ๊ธฐ๋ฐ์ผ๋ก ์์ฑ๋์์ต๋๋ค. (์ถ์ฒ: PyBAMM) ํ์๋ NMC ์ ํ๋ผ๋ฏธํฐ ์ ์ ๋ํ ์ ๋ณด๋ฅผ ํ์ ํ๊ณ ์ OKane2022 paramet..
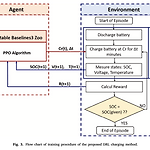
๐ MSCC-DRL: Multi-Stage constant current based on deep reinforcement learning for fast charging of lithium ion batteryJournal of Energy Storage, 2024 ์ ๊ธฐ์ฐจ(EV) ์์ฅ์ ๊ธ์ฑ์ฅ๊ณผ ํจ๊ป ๊ทนํ ๊ธ์ ์ถฉ์ (Extreme Fast Charging, XFC)์ ๋ํ ํ์์ฑ์ด ์ ์ ๋ ์ปค์ง๊ณ ์์ต๋๋ค. ํนํ, ๋ฐฐํฐ๋ฆฌ๋ฅผ 10~15๋ถ ๋ด์ 80% SOC(State of Charge)๊น์ง ์ถฉ์ ํ๋ ๊ฒ์ด ์ ๊ณ์ ์ฃผ์ ๋ชฉํ๋ก ์ค์ ๋๊ณ ์์ต๋๋ค. ํ์ง๋ง ๊ธ์ ์ถฉ์ ์ ์งํํ ๊ฒฝ์ฐ, ๋ฐฐํฐ๋ฆฌ ๋ด๋ถ ์ํ๊ฐ ๊ธ๊ฒฉํ๊ฒ ๋ณ๋ํ๋ฉด์ ๋ฐฐํฐ๋ฆฌ์ ์ดํ๋ ๋น ๋ฅด๊ฒ ์งํ๋ ์ ์์ต๋๋ค. ๋ฐ๋ผ์, ๋ฐฐํฐ๋ฆฌ์ ์ดํ๋ฅผ ์ต์ํํ๋ฉด์๋ ๋น ..
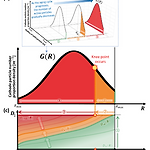
๐ Electrochemicalโmechanical coupled model for computationally efficient prediction of long-term capacity fade of lithium-ion batteriesJournal : Journal of Energy Storage โ24 (IF 8.9) [ summary ]์ ์ ๋ชจ๋ธ : ISIF model, SVD-ISIF modelAging์ ์ํฅ์ ์ฃผ๋ mechanical fatigue fracture of cathode particles ๋ชจ๋ธ๋งISIF ( inhomogeneous stress-induced fracture)Electrochemicalโmechanical coupled capacity fade modelKne..
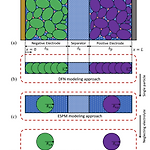
๋ค์์ 24๋ ๋ 2ํ๊ธฐ ๋ฐฐํฐ๋ฆฌ ๊ฐ์๋ฅผ ๋ค์ผ๋ฉฐ ์์ฑํ ๋ ํฌํธ์ด๋ค. Li-ion ๋ฐฐํฐ๋ฆฌ์ electrochemical model ์ค DFN, SPMe, SPM์ ์ค์ ์ ์ผ๋ก ๊ฐ ๋ชจ๋ธ์ ์์์ ๋ํด ์์ ํ์์ผ๋ฉฐ, ๊ฐ ๋ชจ๋ธ์ computational cost์ accuracy์ trade-off ๋ฅผ ์ค์ ์ ์ผ๋ก ์กฐ์ฌํ์๋ค. Abstract๋ณธ ๋ณด๊ณ ์๋ ๋ฆฌํฌ์ด์จ ๋ฐฐํฐ๋ฆฌ์ electrochemical ๋ชจ๋ธ์์ ์ค์ํ ์ญํ ์ ํ๋ DFN(Doyle-Fuller-Newman)๊ณผ DFN์ simplified model์ธ SPMe(Single Particle Model with Electrolyte), SPM(Single Particle Model)์์ ์ฌ์ฉ๋๋ ์ฃผ์ ๊ฐ๋ ๊ณผ ํน์ฑ์ ์ค์ฌ์ผ๋ก ์์ ํฉ๋๋ค. ํนํ DFN, SP..
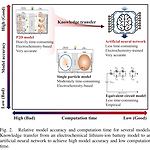
Capacity Estimation of Lithium-Ion Batteries for Various Aging States Through Knowledge TransferJournal : IEEE Transactions on Transportation Electrification โ21 (IF 7.2)17 citations [ summary ]electrochemical knowledge๋ฅผ ํ์ตํ๋ inverted bottleneck network(IBN)์ ๊ธฐ๋ฐ์ผ๋ก ๋ฆฌํฌ ์ด์จ ๋ฐฐํฐ๋ฆฌ์ aging states์ ๋ํ ์๋ก์ด capacity estimation ๋ฐฉ์ ์ ์๋ฌผ๋ฆฌ์ ์ผ๋ก ๊ฐ๋ฅํ ํ๋ผ๋ฏธํฐ ์กฐํฉ์ ๋ํด sythetic data ์์ฑ (๋ฐฐํฐ๋ฆฌ์ ํน์ฑ ์ ๋ณด๋ฅผ knowledge transfer) โ AE..
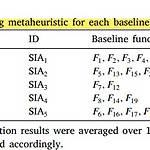
Strategically switching metaheuristics for effective parameter estimation of electrochemical lithium-ion battery modelsJournal : Energy Storage โ23 (IF 8.9)10 citations [ summary ]SIA์ 23๊ฐ baseline function ์ค 10๊ฐ ์ ํ (ํน์ metaheuristic์ ํจ๊ณผ์ ์ธ ๋ชจ์) (table 2)10๊ฐ์ baselie function ํ์ตํ FuncNet(CNN)์ ์ฌ์ฉํด object function์ ๊ฐ์ฅ ์ ์ฌํ baseline function ์๋ณ โ FuncNet์ผ๋ก objective function identifyํจCNN์์ ์๋ณ๋ ๊ฐ์ฅ ์ ์ฌํ..
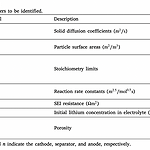
Effective and practical parameters of electrochemical Li-ion battery models for degradation diagnosis Journal : Energy Storageโ 21 (IF 8.9)32 citations [Summary]params ์ค์๋ fixed params, dynamic params๊ฐ ์์15๊ฐ์ dynamic params๋ aging์ ๋ฐ๋ผ ๋ณํจ15๊ฐ์ params์ ๋ํด GA ์๊ณ ๋ฆฌ์ฆ์ผ๋ก ํ๋ผ๋ฏธํฐ ์ต์ ํ15๊ฐ์ params ์ค์ battery degradation diagnosisํ๋ ํ๋ผ๋ฏธํฐ ์ ํ3๊ฐ์ง ์๋ ด ์กฐ๊ฑด , CI (Confidence Interval) ์ฌ์ฉ๊ฒฐ๊ณผ : degradation diagnosis ํ๋ผ๋ฏธํฐcat..
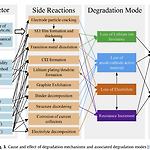
๐ A review on the key issues of the lithium ion battery degradation among the whole life cycle eTransportation 1 (2019)citations : 1009 [ ๊ฐ๋จ ์์ฝ ]์ด ๋ ผ๋ฌธ์ ๋ฆฌํฌ ์ด์จ ๋ฐฐํฐ๋ฆฌ์ ์ ์ฒด ์๋ช ์ฃผ๊ธฐ์ ๊ฑธ์น ์ดํ(degradation) ์ด์๋ฅผ ์ข ํฉ์ ์ผ๋ก ์ ๋ฆฌํ ๋ฆฌ๋ทฐ ๋ ผ๋ฌธ๋ฐฐํฐ๋ฆฌ ๋ด๋ถ ์ดํ ๋ฉ์ปค๋์ฆ์ ๋ํ ์ค๋ช - ์๊ทน/์๊ทน ์ฌ๋ฃ๋ณ ์ดํ ์์ธ ๋ถ์๋ฐฐํฐ๋ฆฌ ์ดํ์ ์ํฅ์ ๋ฏธ์น๋ ์์ธ๋ค์ค๊ณ(design) ๋จ๊ณ์์์ ์ํฅ: ์ฌ๋ฃ, ์ ๊ทน, ์ , ์์คํ ๋ ๋ฒจ ์ค๊ณ์ ์กฐ(manufacture) ๋จ๊ณ์์์ ์ํฅ: ์ ๊ทน ์ ์, ์ ์กฐ๋ฆฝ, ์ ํด์ง ์ฃผ์ , ํ์ฑ ๊ณต์ ๋ฑ์ฌ์ฉ(application) ๋จ๊ณ์์์ ์ํฅ: ์จ๋, S..